It is all about integraition of different technologies
Feature labeling.
Macro photography
And this is our case.
Before exploring the technologies, let’s have a look how skin cancer pattern recognition is done by humans.
The Cells
Melanocytes
Why are they so different from other skin cells?
Special kind of skin cells that produce melanin – dark pigment that protects our skin from the sun radiation.
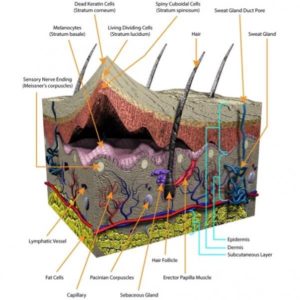
What is Skin Cancer
DNA damaged cells
At the same time melanocytes are source of the most dangerous form of skin cancer – Melanoma.
Skin cancer cells are DNA damaged cells that, unlike other form of cancer, are visible under certain conditions.
Taking picture
Dermatoscopy
Usually, doctors take a tissue picture with Dermatoscope, special equipment with magnifying glass that allows to make macro photo in natural and polarized light with different scales and filters.
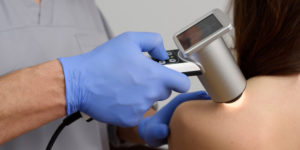
Picture analysis
Risk Assessment
Then image is labeled based on one of the pattern recognition methods. Some of the most popular are: ABDCE, Seven Point Check-list, Menzies methos. All of them are slightly different, but all of them use the same visual color, structure and pattern recognition techniques.
HOW TECHNOLOGIES CAN HELP?
The Core Technology
Convolutional Neural Network
At the heart of our skin cancer detection system lies Convolutional Neural Networks (CNNs), a class of deep learning models tailored for image recognition tasks. CNNs excel at analyzing visual data, making them particularly effective for medical image analysis, such as identifying skin abnormalities. By training our CNN on thousands of dermoscopic images of both benign and malignant lesions, our technology learns to differentiate between healthy skin and potential cases of skin cancer, including melanoma.
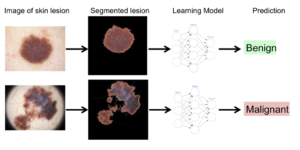
Evolution
Self learning
Our CNN model processes images by breaking them down into layers, analyzing features like color, texture, and patterns that are indicative of skin cancer. The network identifies subtle differences in visual markers, which may be hard for the human eye to detect, and applies this knowledge to classify new, unseen images.
Over time, with continuous training and improvements, our system becomes even more adept at recognizing complex and rare skin cancer cases, ensuring higher accuracy and reliability.
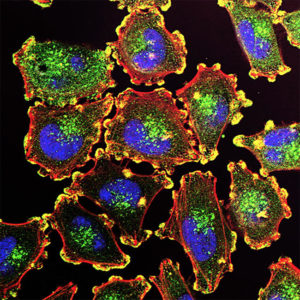
WHAT ABOUT TRAINING DATA?
Training
Data sets
To enhance the accuracy and robustness of our skin cancer detection system, we train our CNNs using a variety of data sources, a mix of public datasets, clinical data, and crowdsourced images.
Good to start
One of the largest skin cancer image datasets is collected by The International Skin Imaging Collaboration (ISIC) and it keeps 482,781 images.
About ISIC
ISIC focuses on creating and sharing a vast repository of dermoscopic images, which are used by researchers and medical professionals to advance skin cancer detection.
By fostering collaboration among dermatologists, researchers, and AI experts, ISIC helps to promote the development of accurate, AI-driven diagnostic tools. Their efforts are critical in enhancing early detection and treatment of skin cancers, including melanoma, worldwide.
Good for labelling and calibration
Pilot testing
For precision
More training data
Data quality
Calibration and mapping
HOW TO GET DERMATOSCOPY IMAGE?
Device
Dermatoscope
A dermatoscope is a medical device used for examining skin lesions with enhanced magnification and illumination. It allows dermatologists to observe the skin in greater detail, revealing structures beneath the surface that are not visible to the naked eye.
By using polarized or non-polarized light, dermatoscopy helps in diagnosing skin conditions like melanoma, basal cell carcinoma, and other skin abnormalities.
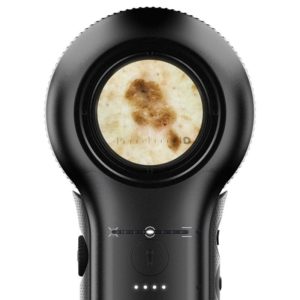
Can you do it with you Iphone?
Macrophotography
In 2021, Apple released the iPhone 13 Pro with advanced lens technology that enabled macro photography, setting a new standard for flagship models across other smartphone brands. Modern smartphone cameras now feature lenses and sensors capable of capturing high-quality close-up images with exceptional detail.
With the right lighting conditions, these cameras can produce skin images of sufficient quality to support dermatoscopic assessments. Combined with specialized apps and AI-driven tools, this technology allows for convenient documentation and analysis of skin conditions, offering an accessible, portable alternative to traditional dermatoscopes for both users and healthcare professionals.
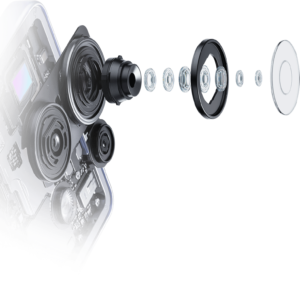
WE ARE EXCITED ABOUT THE OPPORTUNITY TO CREATE CONVENIENT TOOL FOR SKIN CANCER ASSESSMENT